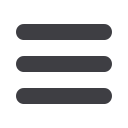
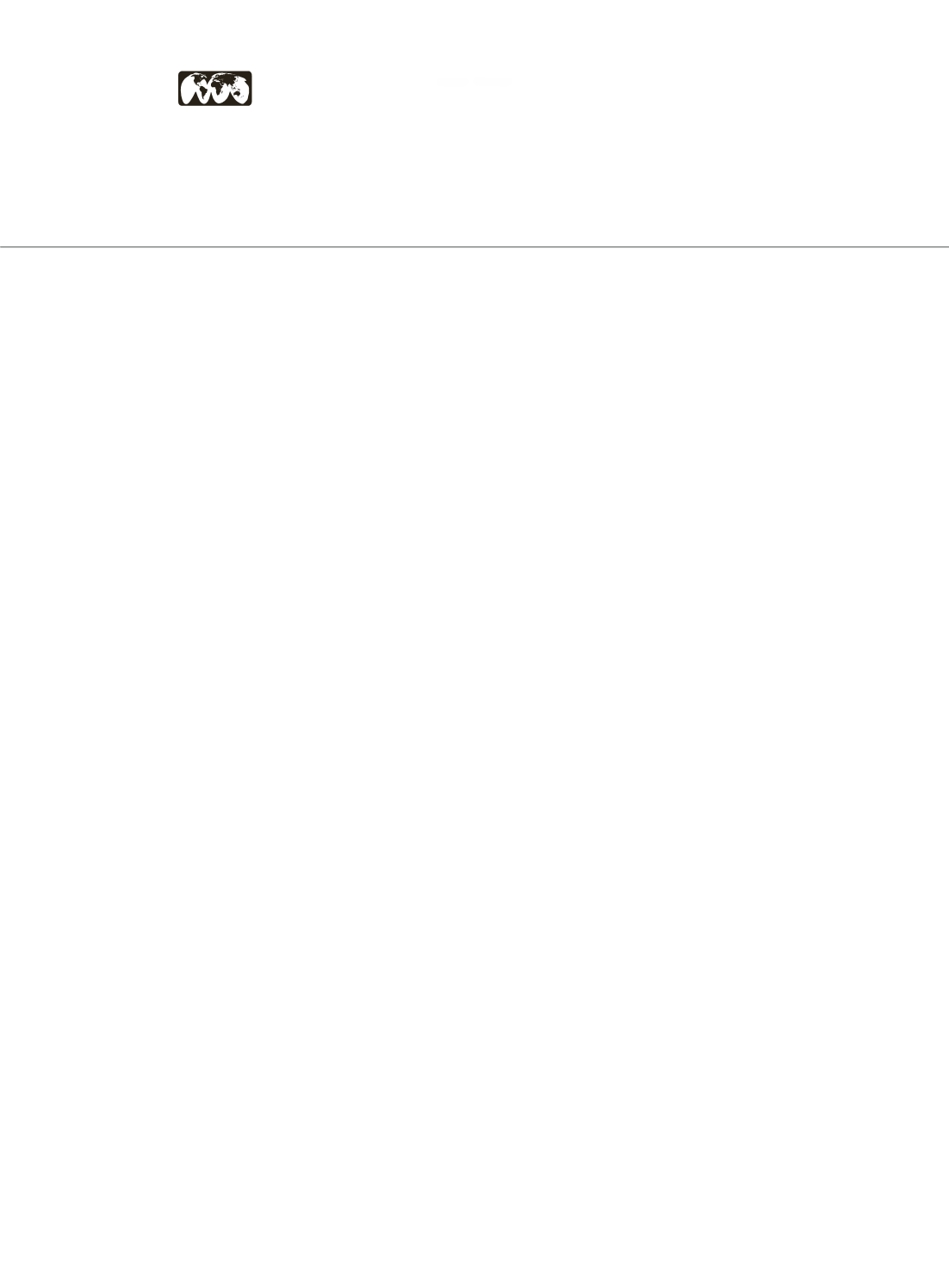
allied
academies
Page 32
June 12-13, 2019 | Edinburgh, Scotland
8
th
European Clinical Microbiology and Immunology Congress
&
3
rd
World congress on Biotechnology
Joint Event
Microbiology: Current Research | Volume: 3 | ISSN: 2591-8036
Efficacy analysis of acid-fast bacillus detection for tuberculosis by smart medical microscope
imaging system
Hui-Zin Tu
1,2
, Chii-Shiang Chen
1
, Huei-Cin Sie
1
, Tsi-Shu Huang
1
, Susan Shin-Jung Lee
1
and
Herng-Sheng Lee
1
1
Kaohsiung Veterans General Hospital, Taiwan
2
National Kaohsiung Normal University, Taiwan
Background
: Tuberculosis is an emerging infectious disease
worldwide. The most robust and economical method,
recommended byWHO, for first line laboratory diagnosis of
pulmonary tuberculosis is acid-fast stainmethod of sputum
smears for acid-fast bacilli (AFB) detection. However, it
mostly relies on artificial microscopic examination, which
may be tedious. The use of such an automated systemmay
significantly increase the sensitivity of bacilli detection.
The objective of this study is to adopt an automated
system for identification of AFB under microscope using
image recognition technology.
Method
: The study was carried out in Kaohsiung Veterans
GeneralHospital,Taiwan.Anautomatedmicroscopesystem
(“system”) (TB-Scan 1.0, Wellgen Medical, Kaohsiung) was
used in the TB laboratory. The system consists of two
components: (1) Microscopic imaging acquiring hardware
with auto-focusing and slide-scanning mechanism to cover
the specimen based on WHO recommendation (300 fields
with 100x oil immersion); (2) Image recognition software
for detection and classification of positive AFB in images.
The microscopic images were digitally captured and stored.
In the detection phase, candidate AFBs were marked and
differentiated from other substances in smear based on
color and morphological features. In the classification
phase, the feature parameters were extracted from AFB
candidates as the input parameters to a proprietary
classifier. The result was recorded as positive if any AFB
was identified in the image of the slide. We used the results
with medical technicians as gold standard in evaluating the
system performance. Slides with incomplete stain removal
and inconsistent viewing fields were excluded from the
study (<3%).
Results
:When the systemwas installed in July 2017, the first
test results (from July to September of 2017, n=1,050) was
not satisfactory. The sensitivity and specificity were only
13.3% (2/15) and 7.9% (73/925), respectively. After a series
of customized imaging training and testing, the second test
results (from October to December of 2017, n=2,254) were
slightly improved: The sensitivity was 28.8% (34/118) and
specificity was 53.8% (1,105/2,053), respectively. However,
if technicians can be involved in assisting confirming the
images to rule out the false-positives along-side with the
automated system, the accuracy, sensitivity and specificity
can be further improved to 93.0% (2,096/2,254), 67.8%
(137/202) and 98.4% (1,959/1,991), respectively. At
manufacturer continuous image training by machine
learning algorithms, the performance had incremental
improvement. For February 2019 only results (n=448),
the accuracy, sensitivity and specificity were stability to
91.7% (411/448), 66.2% (43/65) and 96.3% (368/382),
respectively.
Discussion
: To our knowledge, this is the first of such
automated microscope system for TB smear testing in a
control trial. Although the performances of the system still
have room for improvement, the following issues are worth
considering: (1) Medical technicians as gold standard in this
study were applied. The system, for example, detected 135
smears positive for AFB but missed by technicians initially
but later the final results were reviewed and retrofitted.
The result comparisons (technician vs. culture and system
vs. culture) may provide more information about the
system’s performance; (2) A continuous and customized
image training for optimizing recognition performance by
machine learning is the key to success. TB smear detection
is not “one system fits all” and customized training at each
laboratory is essential; (3) The inter-laboratory and intra-
laboratory variables could compromise the performance
of such system. For example, machine staining would be
more consistent compared to manual staining; (4) The
objective of the automated system is to increase the
test performance and not meant to replace laboratory
technicians. Experienced technicians are still needed to
further improvement of the system.
Hui-Zin Tu et al., Microbiol Curr Res, Volume 3
ISSN: 2591-8036